Is your AI good? Not if your data is bad.
- Shannon Harney
- Aug 19, 2021
- 2 min read
According to a recent study from MIT, 10 of the most commonly-used computer vision datasets have errors that are “numerous and widespread” -- and it could be hurting your AI.
Data sets are core to the growing AI field, and researchers use the most popular machine-learning models to evaluate how the field is moving forward. These include image recognition data sets like ImageNet or MNIST, which focuses on the ability to recognize handwritten numbers between 0 and 9.
But… the most popular AI data sets have some major problems.
Even these popular and long-standing data sets have been shown to have major errors.
For ImageNet, this included huge privacy problems when it was revealed that they had used people’s faces in their data set without their consent.
MIT’s most recent study looks at a more mundane, but possibly more important, problem -- annotated data labels are completely wrong. A couch is labeled as a kayak, a house as a marmot, or a warehouse pallet as a Honda Civic. For ImageNet, the test set had an estimated error rate of over 5%, and for QuickDraw (a compilation of hand drawings), the rate was over 10%.
How’d the MIT study work?
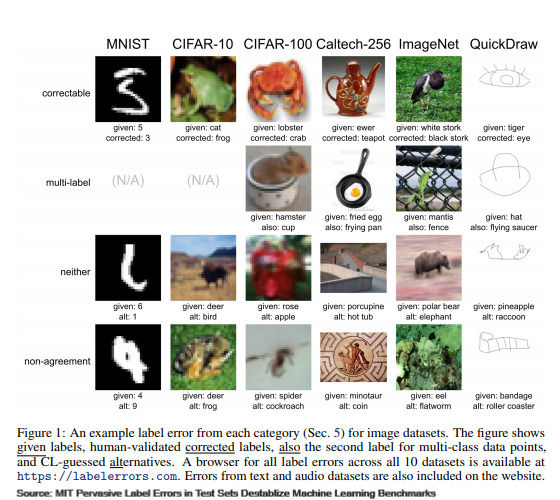
The study used 10 of the most popular data sets with a matching set for validation. The MIT researchers basically built their own machine-learning model and used it to predict the labels in the testing data. Where they disagreed, that piece of data was flagged for review and five Amazon Mechanical Turk human reviewers voted on which label they thought was correct.
What does this mean in the real world?
The data people are using to train their AIs isn’t any good so their models are suffering.
The research examined 34 models previously evaluated against an ImageNet test sets, and then evaluated them again against the 1,500 or so examples where the data labels were wrong. And it turned out that the models that didn’t do well on the original, error-filled data set performed among the best once those labels were fixed.
And the simpler models, with correct data labels, performed far better than more complicated models used by giants in the industry like Google -- the things everyone assumes to be the best in the field.
Basically, these advanced models are doing a bad job -- and it’s all because the data is bad.
So how do I fix my data problems? Lexset synthetic data.
Here at Lexset, we create synthetic training data for your AI -- and our data labels are always correct.
You can create infinite datasets with pixel-perfect annotation, enabling rapid model iteration, advanced bias controls -- all leading to dramatically improved accuracy.
Whether you’re trying to count, measure, monitor or all of the above, our synthetic data makes it easy to train your AI to do the right thing, every time.
Ready to get started? Reach out now and we’ll have you on your way to perfect data annotations in no time.
Note: The 10 datasets examined are MNIST, CIFAR-10 / CIFAR-100, Caltech-256, ImageNet, QuickDraw, 20news, IMDB, Amazon Reviews, AudioSet
コメント